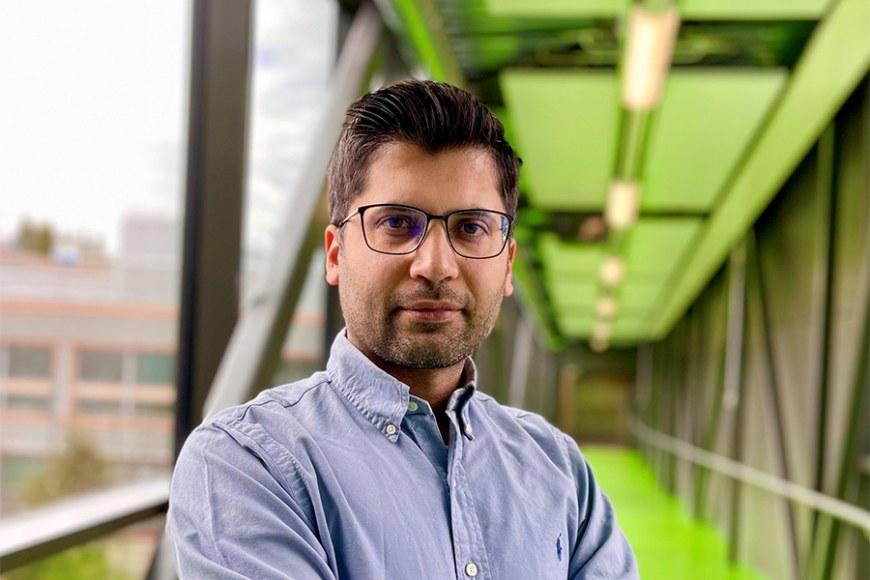
In medical diagnosis, the data from non-healthy subjects are either hard or simply impossible to obtain. For example, in mammography for cancer detection, the specific target class recognition of cognitive brain functions, in the interstitial lung diseases categorization, or detecting nosocomial infections through clinical data, a representative training set representing also non-healthy cases is challenging to obtain. In such cases, one-class classification methods are used to create a model.
In Sohrab’s proposed subspace learning approach for one-class classification, the aim is to transform the features from a given space into a lower-dimensional space optimized for better classification accuracy. Sohrab also developed a method for optimizing subspace in the case of multimodal data, where the same object is represented by several different feature vectors (e.g., image and sound sample).
The capability of one-class classification methods to improve the performance of a deep convolutional neural network to identify rare benthic macroinvertebrates is also demonstrated in the thesis.
“Unavailability of data from one or several categories led to the inception of machine learning methods that require data only from one class during the training process. One-Class Classification methods are used to create a model for predicting whether an unseen sample comes from this class of interest. For example, to train a model for anomaly detection, it is usually challenging to collect anomalous data for training, but the normal data is available in abundance,” states Sohrab.
His thesis provides a new paradigm for creating one-class classification models which can be used for situations where it is vital to identify one of the categories, but the examples from that specific category are scarce.
The doctoral dissertation of Fahad Sohrab in the field of machine learning titled Subspace Support Vector Data Description and Extensions will be publicly examined in the Faculty of Information Technology and Communication Sciences at Tampere University at 14 o’clock on 27 May 2022 in auditorium TB109 of the Tietotalo (Korkeakoulunkatu 1, Tampere). Dr. Hichem Sahbi from Sorbonne University in France will serve as the Opponent during the thesis defense. The Custos will be Professor Moncef Gabbouj from Tampere University. The thesis is co-supervised by Dr. Jenni Raitoharju, Tampere University and Finnish Environment Institute Finland.
The dissertation is available online at the https://urn.fi/URN:ISBN:978-952-03-2409-4
Photo: Zeeshan Waheed